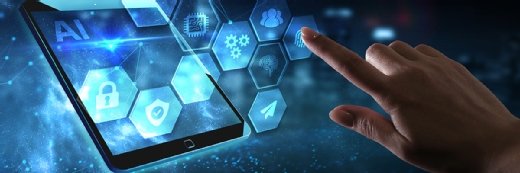
putilov_denis - stock.adobe.com
Customer segmentation analytics evolve with GenAI, ML
GenAI, machine learning and advanced analytics techniques automate time-consuming aspects of customer segmentation, freeing up analysts to find insights and create value.
Advanced analytics models and machine learning algorithms power customer segmentation strategies. Automation advancements in AI can lead to faster, higher-quality results.
Customer segmentation enables organizations to tailor messages -- from sales pitches to outreach campaigns -- to groups of seemingly similar individuals. It groups people together based on shared characteristics, such as age, gender, income, interests, location and spending habits.
Segmenting customers helps minimize the time and cost of developing on-point messages, while maximizing the effectiveness of engaging the recipients. As organizations look toward personalization with the goal of tailoring services to every individual, customer segmentation remains a valuable and important practice. Today, some customer segmentation tools have new advanced analytics, machine learning (ML) and generative AI (GenAI) capabilities.
"Segmentation is not going anywhere," said Zeid Khater, analyst at Forrester Research. "There's not a huge shift away from it. And most [organizations] are asking how to make the best use of it."
Customer segmentation analytics uses
The principle behind segmentation is that grouping individuals according to shared traits provides insight into what matters to them collectively and what resonates with them as individuals. Organizations can gain customer insights without the more complex and costly work of getting to know them one by one.
Organizations use the insights generated from customer segmentation analysis to target groups with appropriate messages, such as advertising and marketing campaigns, and relevant appeals.
"This ability to do customer segmentation allows you to decide where you want to put the money," said Rod Fontecilla, who served as partner and chief innovation officer at the consultancy Guidehouse until March 2024.
Marketing professionals are the most common practitioners of customer segmentation, but product developers, as well as social, political and charitable organizations, use segmentation, too.
"Segmentation can be used anyplace where you have data sets representing people and conditions," said Thomas Coughlin, president of consulting firm Coughlin Associates.
The desire and ability to segment and group individuals by shared characteristics predates computers, but technology can segment and group customers at a scale and speed that humans cannot match. Modern segmentation analysis requires a large amount of data and typically uses one of several analytical methods.
One of the most common methods is k-means clustering, which uses ML algorithms to divide observations into clusters. Analysts specify the number of clusters or segments used in the sorting.
"It's just grouping customers who have similar attributes that make the most sense," Khater said, noting the algorithm doesn't consider the reasons an organization wants to form clusters.
Analysts also use look-alike modeling, another technique that uses ML algorithms to identify and group people based on the demographics of a known group, such as a company's existing customers. Companies use this technique when they want to identify prospective customers, targeting people who are similar to their existing customers, Khater said.
Although the algorithms differ from other common segmentation approaches, experts said segmentation models are probabilistic, not deterministic. The clusters in segmentation analysis are categorized based on a degree of similarity between two or more data sets instead of exact matches.
Segmentation or personalization?
Customer segmentation now faces competition from the drive to personalize. According to the "Customer Loyalty Executive Survey 2023" from PwC, 61% of executives ranked personalizing the customer experience as a high priority -- more than any other loyalty activator. Despite such findings, customer segmentation analytics remain a critical tool.
To start, the analytics work required to fully personalize outreach or services is daunting, Fontecilla said. The cost of personalization work would likely outpace ROI in most, if not nearly all, cases.
Most organizations do not have the data environment required for complete personalization, nor would they want to invest in the requisite computing power, Fontecilla said. Segmentation is easier.
Segmentation can also scale in a way that personalization cannot, and when it's enabled by intelligence and paired with good data sets, segmentation delivers results that provide ROI to organizations.
Dun & Bradstreet use segmentation to support sales, marketing and other functions, said Chief Data and Analytics Officer Gary Kotovets. The analysis helps teams craft campaigns and messaging with an appropriate degree of customization for the targeted group. ML and AI tools in the segmentation technologies improve the accuracy of the groupings.
Additionally, segmentation analytics can deliver increasing amounts of granularity, thanks to advanced analytics and AI, Khater said. Improved capabilities serve as a gateway to personalization, while respecting growing consumer concerns about privacy.
Creating value from customer segmentation analytics
Customer segmentation analytics provide little to no value if an organization does not act based on the identified segments in accordance with business goals.
"Just building a segment to build a segment doesn't work," Khater said. "Understanding the business objective is the best way to do segmentation analysis."
Khater noted that an organization can use the results of its segmentation analysis to identify customers who might be leaving and target them with promotions to keep them in the fold, thereby reducing churn.
Organizations can use analysis results for campaigns to acquire new customers or boost customer retention overall. The data can also fuel and optimize next-best experiences, such as recommendation engines.
Segmentation evolution with advanced analytics, AI and GenAI
Advanced analytics, AI and GenAI bring more value to customer segmentation. Advanced analytics and AI work to improve the quality of the data required for the segmentation analytics. For example, intelligent tools and automation are improving the collection of data from customer surveys and other feedback mechanisms, as well as the analysis of buying patterns and demographic material.
Additionally, AI can create more accurate and more granular groups -- and more of them -- Fontecilla said. AI systems now in use are powerful enough to uncover new patterns among customers. The information that AI and GenAI identify can help fuel predictive analytics models. The new capabilities provide more accurate forecasting of future consumer trends or interests and identify how to prioritize segments.
As GenAI evolves, Fontecilla expects it to eventually help layer more data into the segmentation analysis, helping to bring even more granularity to the process.
"We'll be able to ask questions we could never ask before, and we could get the answers faster," he said.
Mary K. Pratt is an award-winning freelance journalist with a focus on covering enterprise IT and cybersecurity management.